AgroNet ZERO: AI-Driven Data Analysis for Sustainable Agriculture
Harnessing Data Science to optimize soil health and carbon sequestration

Project background
Overview
AgroNet ZERO is developing a data-driven platform to support regenerative agriculture and carbon sequestration through biochar. The platform integrates public and proprietary soil datasets, enabling advanced data analysis to generate soil amendment recommendations for farmers. The system uses machine learning and statistical modeling to analyze carbon impact, soil quality trends, and the effectiveness of biochar applications in different agricultural settings.
The challenge was to build a robust data pipeline capable of aggregating, cleaning, and processing large-scale soil and agricultural datasets while ensuring high accuracy in predictive models. The system needed to provide real-time insights into soil conditions and automate data-driven recommendations for farmers looking to optimize their crop yields and sustainability impact.
Project Goals
- Develop a comprehensive data analysis framework for soil assessment, integrating USDA datasets and proprietary research.
- Build machine learning models to generate prescriptive recommendations for soil improvement and biochar application.
- Implement carbon tracking analytics to measure biochar impact on soil health and climate mitigation.
- Establish an automated data ingestion pipeline for continuous updates and model retraining.
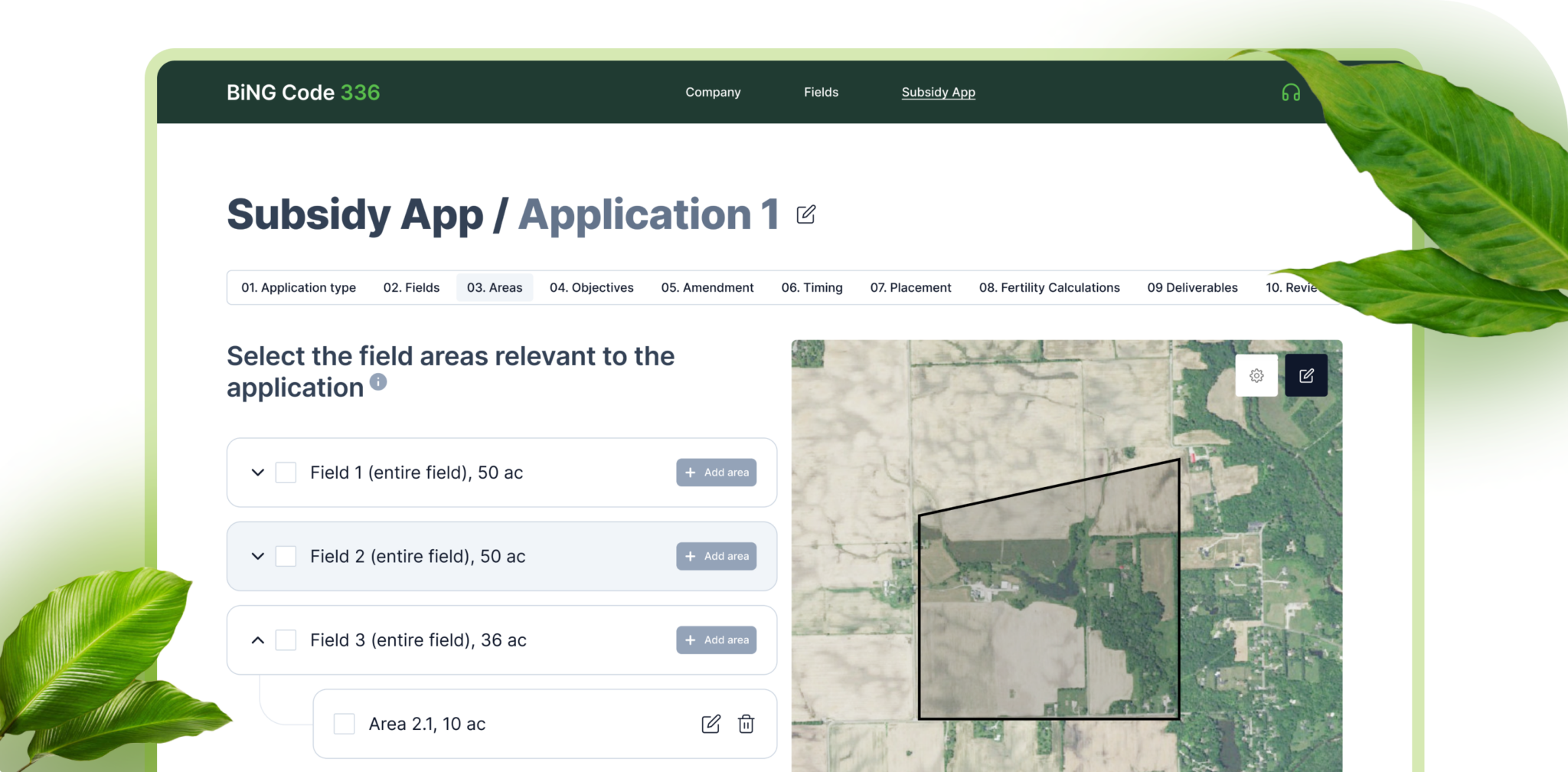
- Webapp
- 5team members
- 500+hours spent
- AI & Analyticsdomain
Challenges
- Integrating structured and unstructured data from USDA Web Soil Survey, field research, and proprietary soil samples.
- Cleaning, validating, and standardizing multiple data sources for reliable analysis.
- Creating predictive models that adjust for different soil conditions, carbon sequestration rates, and climate variables.
- Developing an efficient data pipeline that enables farmers to access up-to-date soil health insights.
Our approach
Solution
Our first step was to build a centralized database that aggregated soil data from multiple sources, ensuring data normalization and consistency. Using Python and Pandas, we developed custom data preprocessing pipelines, handling missing values, outlier detection, and feature engineering for soil-related attributes.
For predictive modeling, we implemented machine learning algorithms using Scikit-learn and TensorFlow, allowing the system to analyze historical soil trends and forecast optimal biochar application for improved carbon retention. The carbon tracking module was built using geospatial analytics, combining satellite imagery with on-ground soil data to measure long-term environmental impact.
For continuous model improvement, we developed an automated training pipeline using Airflow and Docker, retraining models based on newly ingested soil data. A real-time data visualization dashboard was built using Power BI, providing farmers with interactive analytics and soil health reports.
Team
The project was delivered by a cross-functional team of data scientists, machine learning engineers, and data engineers. Data scientists worked on statistical analysis and model development, while data engineers handled large-scale data ingestion and processing. Software developers built the data visualization and reporting tools for seamless user access to insights.
Results
The database now contains a structured repository of agricultural and soil data from multiple states, supporting real-time soil assessment and sustainability tracking. The machine learning models provide high-accuracy recommendations, helping farmers optimize their biochar application and crop rotation strategies.
The automated data pipeline ensures continuous data updates, improving the accuracy of predictive models. The real-time analytics dashboard enables farmers to track soil health metrics and measure the carbon impact of biochar applications, supporting data-driven decision-making in agriculture.
Tools and tech stack
More Projects

- saas
- dotnet
- webapp
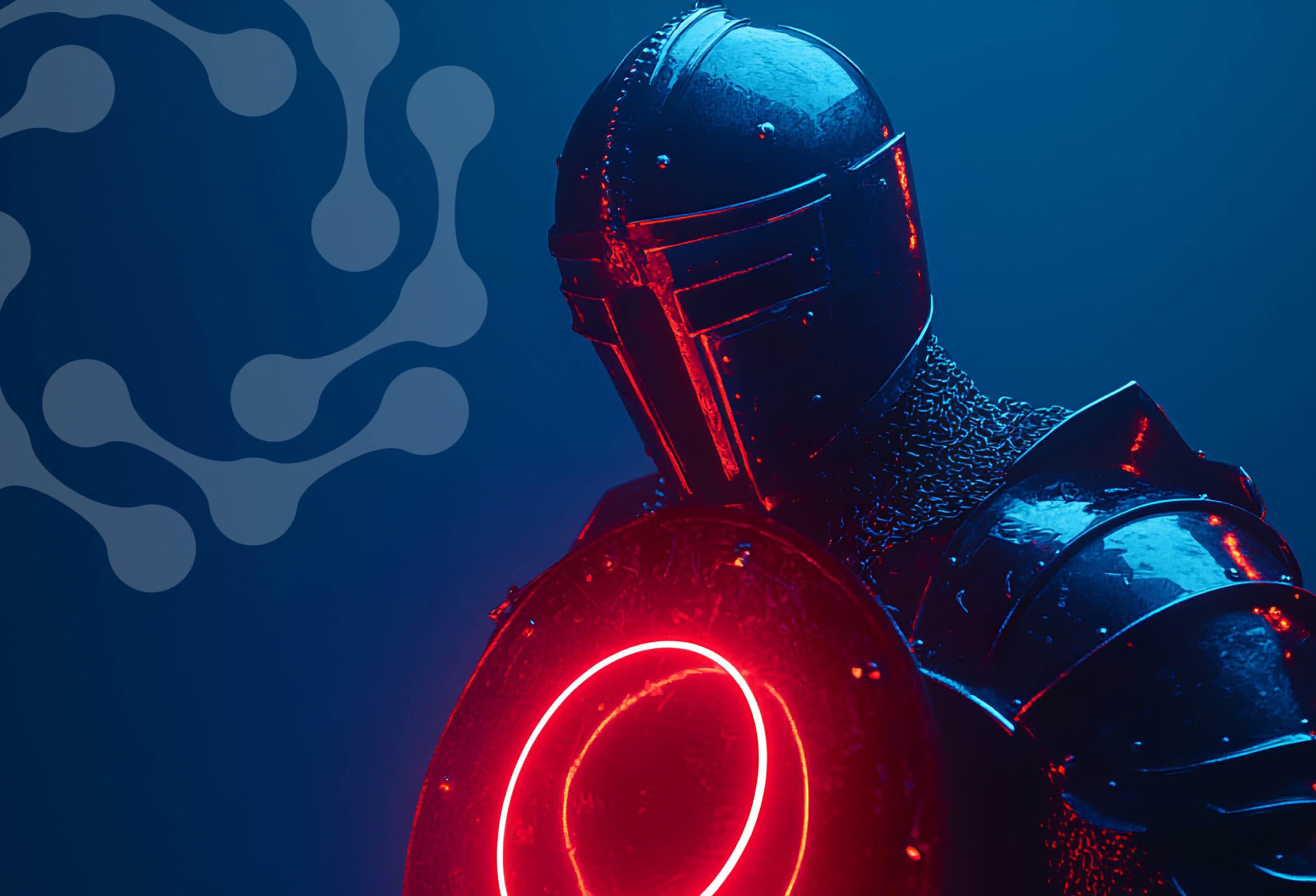
- cybersecurity
- dotnet
- webapp